To make good decisions, it is vital to know the current condition of the field. Core Project 1 develops novel ground and aerial vehicles that operate autonomously and provide precisely georeferenced, phenotypic data from single plant organs over the experimental plot to the field scale. We register 3D structural models of the same plant over time, leading to a 4D reconstruction. Our aim is to develop a new generation of mapping systems as well as a better understanding of the spatio-temporal dynamics of structural and functional plant traits. The goal is to reconstruct several hundred individual plants per day in an experimental field design.
Research Videos

Modern Sensing Applications for Analysing Plant Physiology and Interaction in Mixed Cropping
PhenoRob PhD Student Julie Kraemer talks about her research within Core Project 1 ” 4D Crop Reconstruction” and Core Project 5 “New Field Arrangements”.
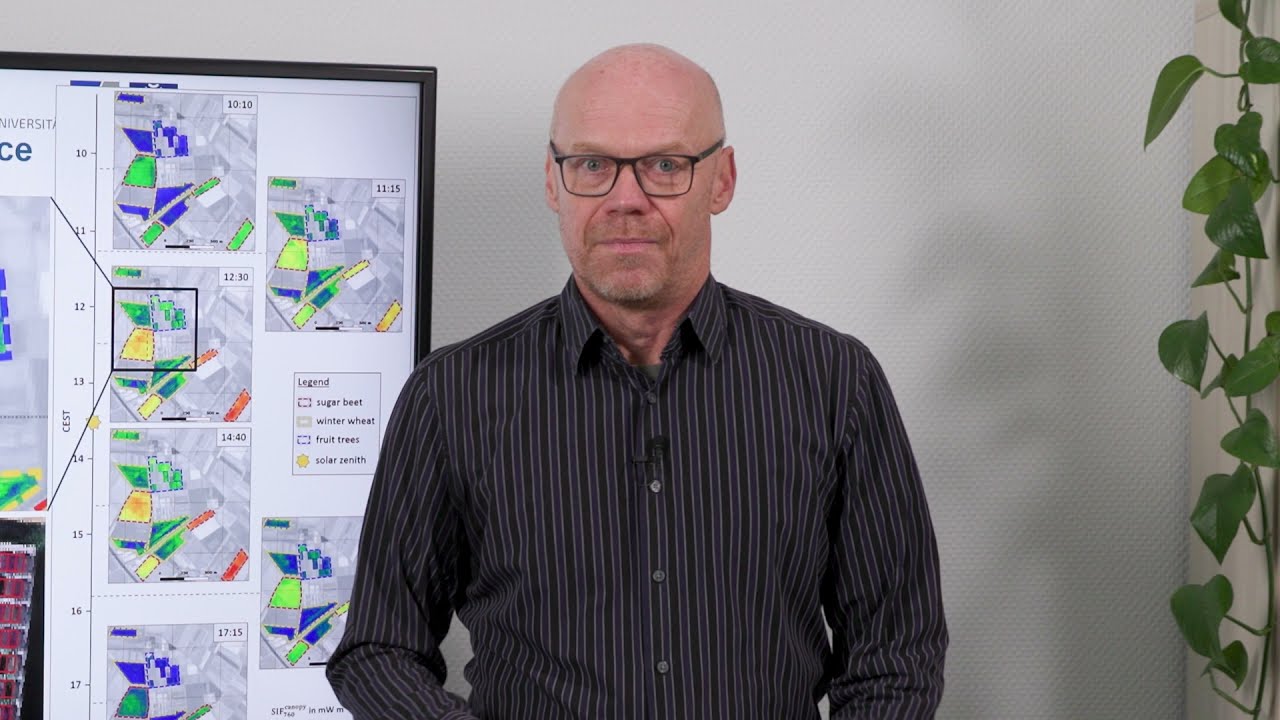
Uwe Rascher: Measuring and understanding the dynamics of plant photosynthesis across scales…
Measuring and understanding the dynamics of plant photosynthesis across scales – from single plants to satellites Prof. Dr. Uwe Rascher is Principal Investigator at PhenoRob and Professor of Quantitative Physiology of Crops, Institute of Bio- and Geosciences (IBG-2), Forschungszentrum Jülich and Institute of Crop Science and Resource Conservation (INRES), University of Bonn Rascher et. al. (2015) Sun-induced fluorescence – a new probe of photosynthesis: First maps from the imaging spectrometer HyPlant Global Change Biology, 21, 4673-4684 https://doi.org/10.1111/gcb.13017 Siegmann et. al. (2019) The High-Performance Airborne Imaging Spectrometer HyPlant—From Raw Images to Top-of-Canopy Reflectance and Fluorescence Products: Introduction of an Automatized Processing Chain Remote Sensing, 11, article no. 2760 https://doi.org/10.3390/rs11232760
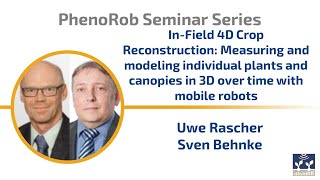
Sven Behnke, University of Bonn and Uwe Rascher, FZJ (11.03.2022)
Sven Behnke (University of Bonn) and Uwe Rascher (FZJ) give a talk on “In-Field 4D Crop Reconstruction: Measuring and modeling individual plants and canopies in 3D over time with mobile robots”
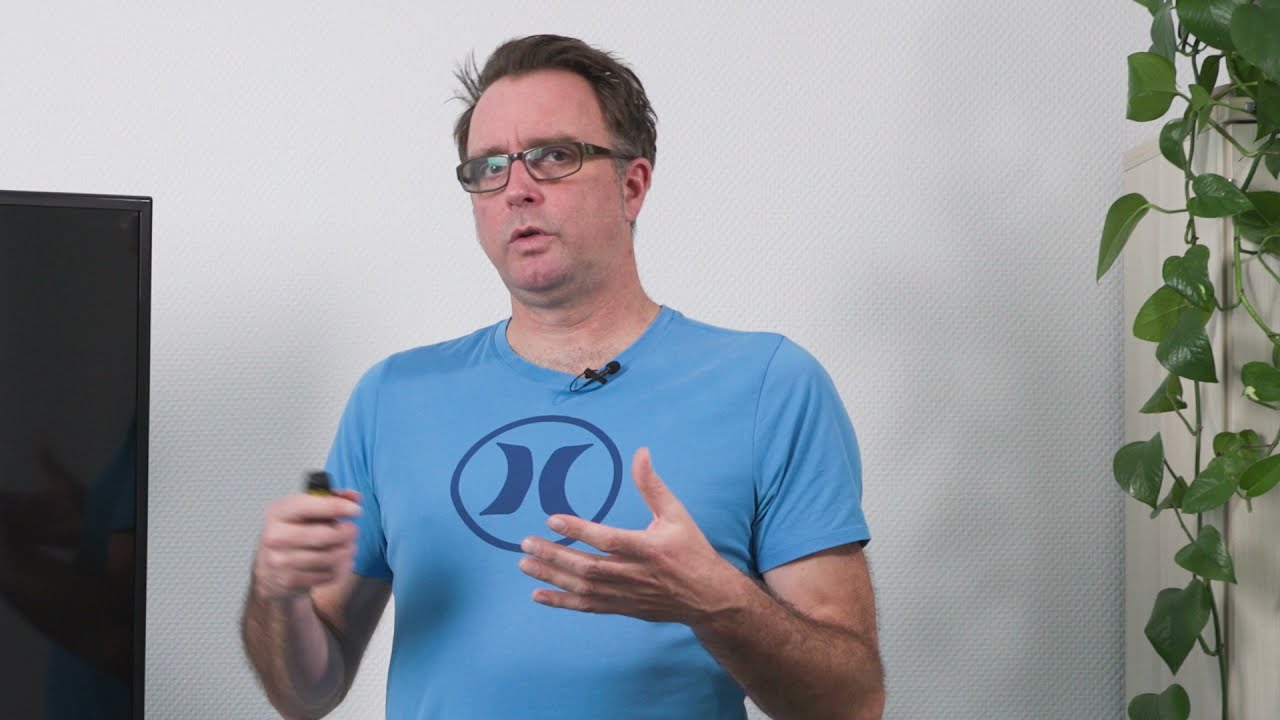
Lasse Klingbeil: Pheno4D: A spatio-temporal dataset of maize and tomato plant point clouds…
Dr. Lasse Klingbeil is Postdoc at the Institute of Geodesy and Geoinformation (IGG), University of Bonn and PhenoRob Member. Pheno4D: A spatio-temporal dataset of maize and tomato plant point clouds for phenotyping and advanced plant analysis D. Schunck, F. Magistri, R. A. Rosu, A. Cornelißen, N. Chebrolu, S. Paulus, J. Léon, S. Behnke, C. Stachniss, H. Kuhlmann, and L. Klingbeil PLOS ONE, vol. 16, iss. 8, pp. 1-18, 2021 Paper: https://doi.org/10.1371/journal.pone.0256340 Data: https://www.ipb.uni-bonn.de/data/pheno4d/
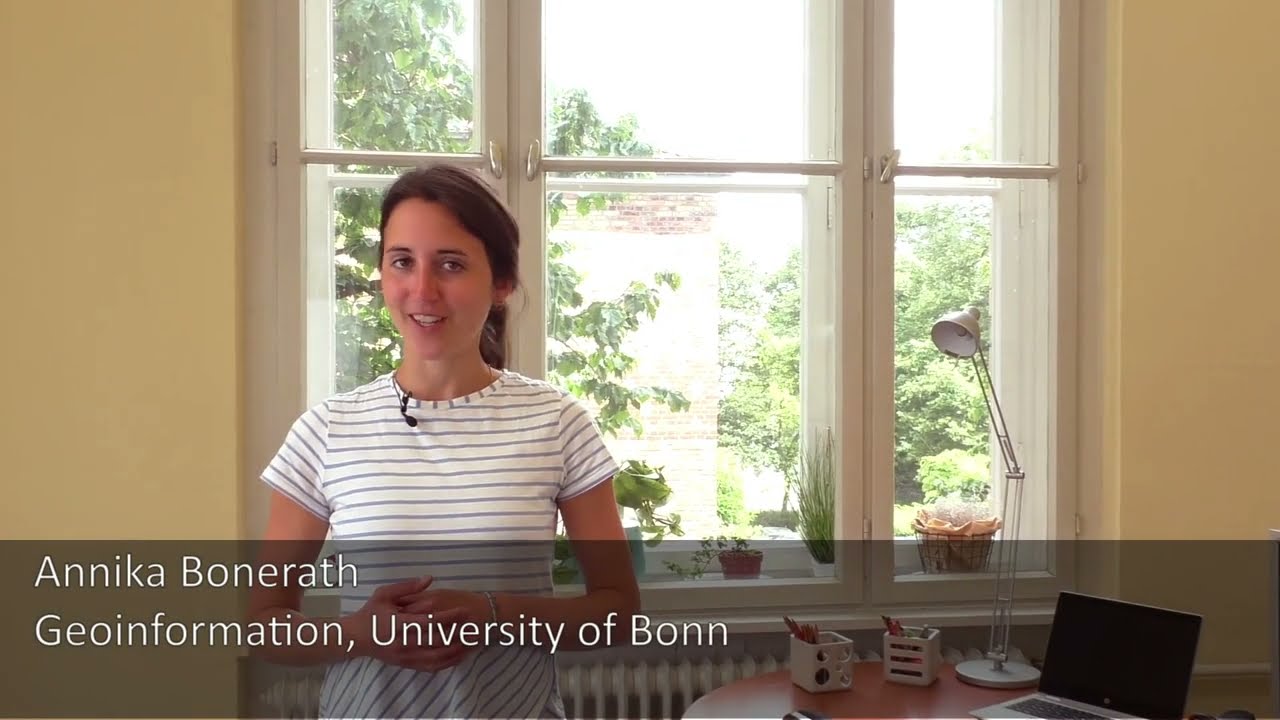
Shortcut Hulls: Vertex-restricted Outer Simplifications of Polygons by A. Bonerath et al.
This short paper trailer video is based on the following publication: A. Bonerath, J. Haunert, J. S. B. Mitchell, and B. Niedermann, “Shortcut Hulls: Vertex-restricted Outer Simplifications of Polygons,” in Proceedings of the 33rd Canadian Conference on Computational Geometry , 2021, pp. 12-23.

LatticeNet: fast spatio-temporal point cloud segmentation using permutohedral lattices (Rosu et al.)
This short paper trailer video is based on the following publication: R. A. Rosu, P. Schütt, J. Quenzel, and S. Behnke, “LatticeNet: fast spatio-temporal point cloud segmentation using permutohedral lattices,” Autonomous Robots, p. 1-16, 2021.
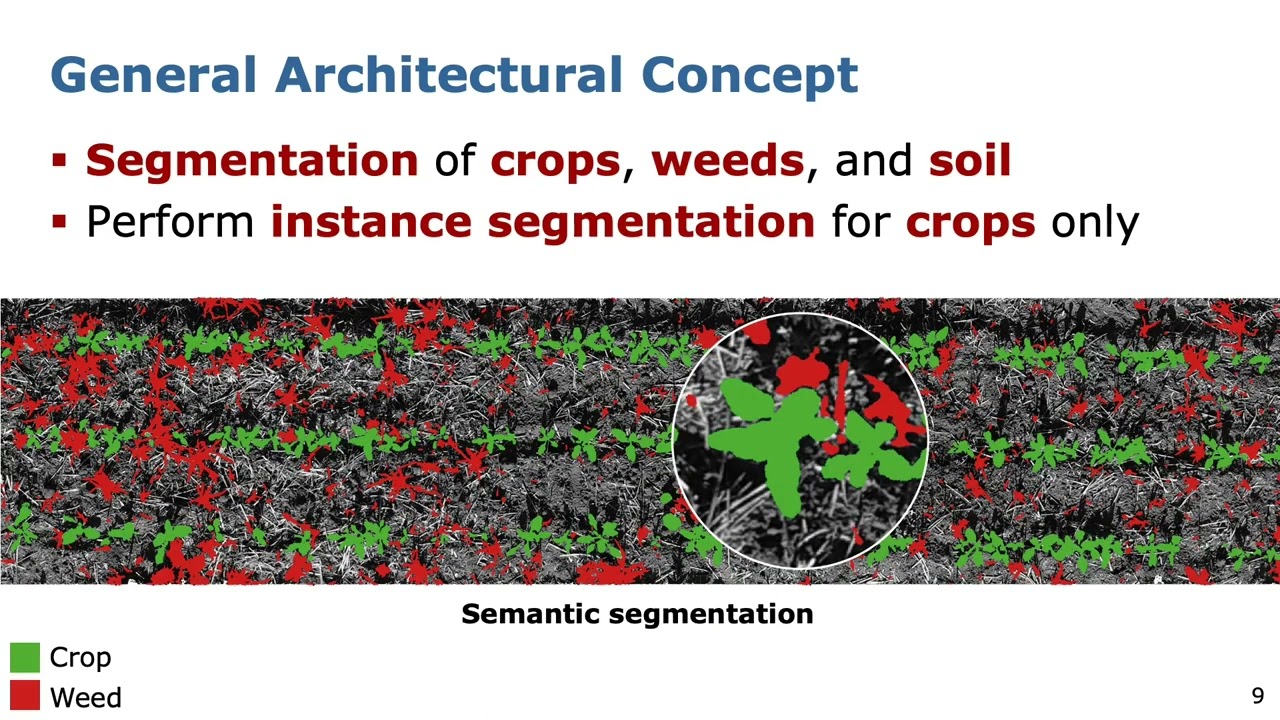
RAL-ICRA’22: Joint Plant and Leaf Instance Segmentation on Field-Scale UAV Imagery by Weyler et al.
J. Weyler, J. Quakernack, P. Lottes, J. Behley, and C. Stachniss, “Joint Plant and Leaf Instance Segmentation on Field-Scale UAV Imagery,” IEEE Robotics and Automation Letters (RA-L), vol. 7, iss. 2, pp. 3787-3794, 2022. doi:10.1109/LRA.2022.3147462 PDF: https://www.ipb.uni-bonn.de/wp-content/papercite-data/pdf/weyler2022ral.pdf #UniBonn #StachnissLab #robotics
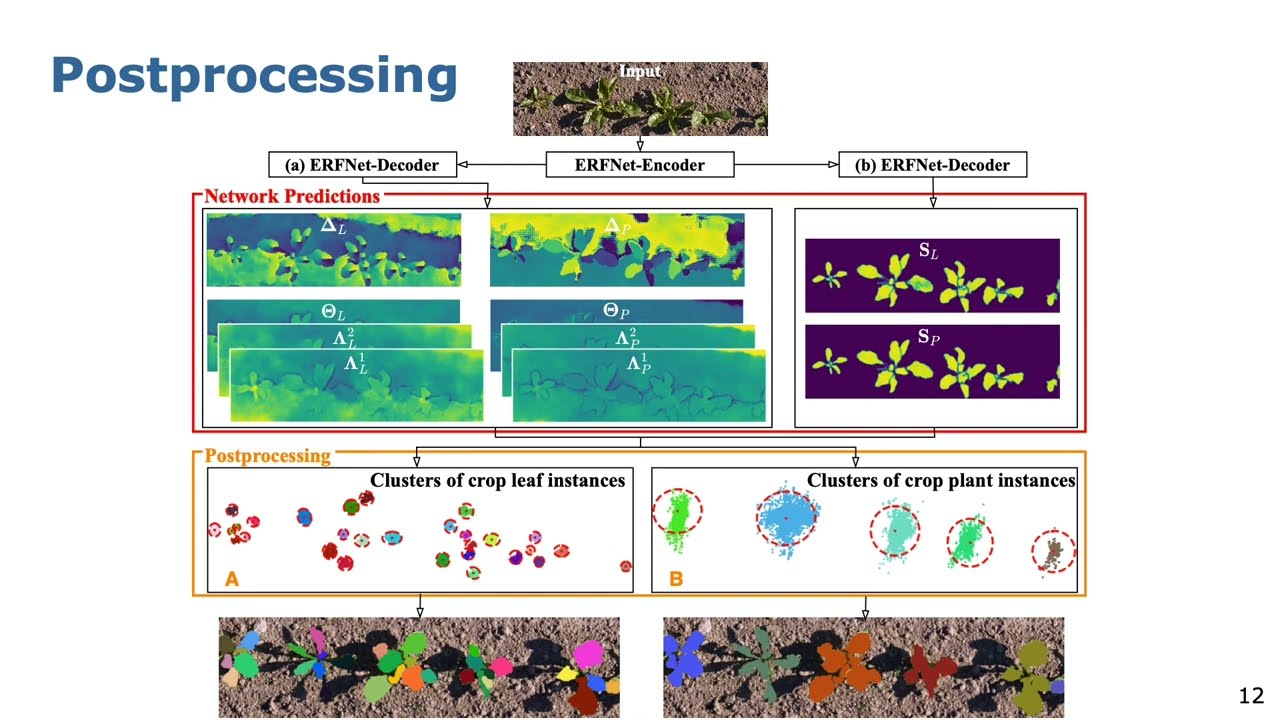
WACV’22: In-Field Phenotyping Based on Crop Leaf and Plant Instance Segmentation by Weyler et al.
J. Weyler, F. and Magistri, P. Seitz, J. Behley, and C. Stachniss, “In-Field Phenotyping Based on Crop Leaf and Plant Instance Segmentation,” in Proc. of the Winter Conf. on Applications of Computer Vision (WACV), 2022. PDF: https://www.ipb.uni-bonn.de/wp-content/papercite-data/pdf/weyler2022wacv.pdf #UniBonn #StachnissLab #robotics

ICRA’21: Phenotyping Exploiting Differentiable Rendering with Consistency Loss by Magistri et al.
F. Magistri, N. Chebrolu, J. Behley, and C. Stachniss, “Towards In-Field Phenotyping Exploiting Differentiable Rendering with Self-Consistency Loss,” in Proceedings of the IEEE Int. Conf. on Robotics & Automation (ICRA), 2021. Paper: https://www.ipb.uni-bonn.de/wp-content/papercite-data/pdf/magistri2021icra.pdf #UniBonn #StachnissLab #robotics #PhenoRob #neuralnetworks #talk
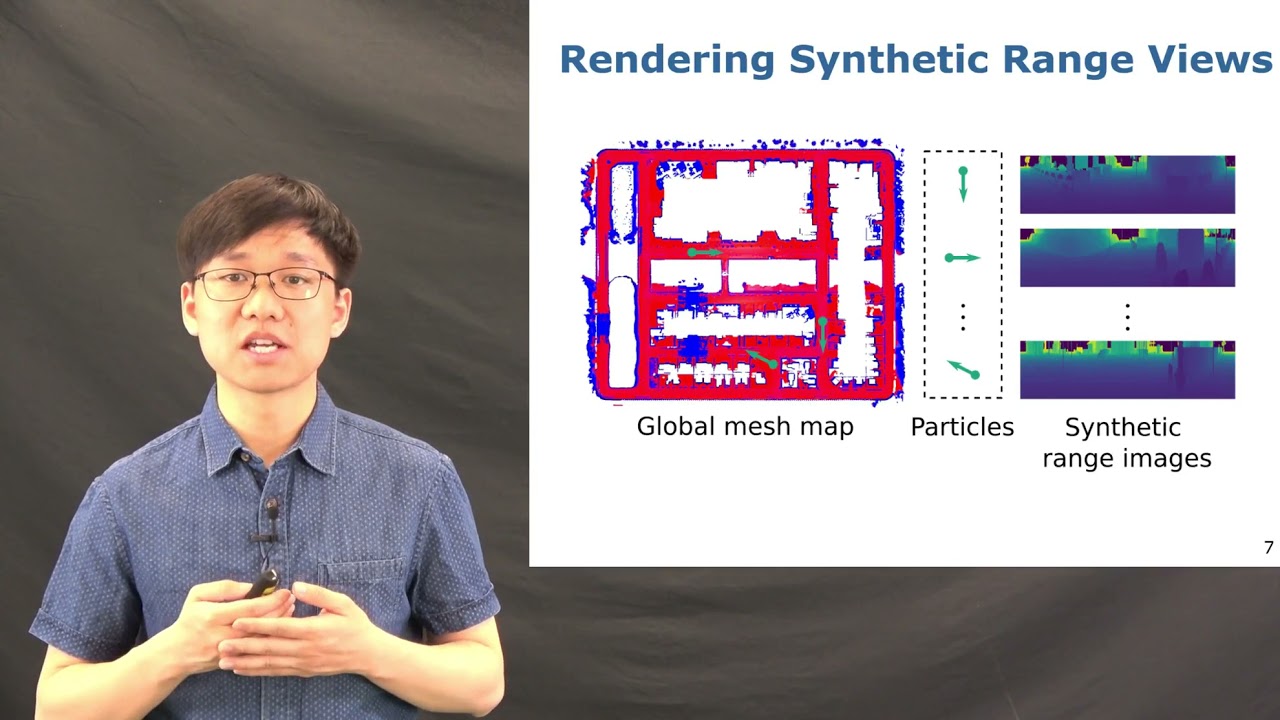
Talk by X. Chen: Range Image-based LiDAR Localization for Autonomous Vehicles (ICRA’21)
X. Chen, I. Vizzo, T. Läbe, J. Behley, and C. Stachniss, “Range Image-based LiDAR Localization for Autonomous Vehicles,” in Proceedings of the IEEE Int. Conf. on Robotics & Automation (ICRA), 2021. Paper: https://www.ipb.uni-bonn.de/wp-content/papercite-data/pdf/chen2021icra.pdf Code: https://github.com/PRBonn/range-mcl #UniBonn #StachnissLab #robotics #autonomouscars #neuralnetworks #talk
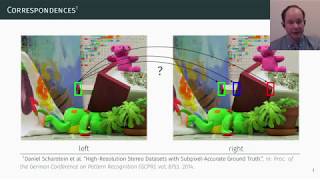
Talk by J. Quenzel on Beyond Photometric Consistency: Gradient-based Dissimilarity for VO (ICRA’20)
ICRA 2020 talk about the paper: J. Quenzel, R. A. Rosu, T. Laebe, C. Stachniss, and S. Behnke, “Beyond Photometric Consistency: Gradient-based Dissimilarity for Improving Visual Odometry and Stereo Matching,” in Proceedings of the IEEE Int. Conf. on Robotics & Automation (ICRA), 2020. PDF: http://www.ipb.uni-bonn.de/pdfs/quenzel2020icra.pdf

IROS’20: Segmentation-Based 4D Registration of Plants Point Clouds for Phenotyping by Magistri et al
F. Magistri, N. Chebrolu, and C. Stachniss, “Segmentation-Based 4D Registration of Plants Point Clouds ,” in Proceedings of the IEEE/RSJ Int. Conf. on Intelligent Robots and Systems (IROS), 2020. Paper: https://www.ipb.uni-bonn.de/wp-content/papercite-data/pdf/magistri2020iros.pdf #UniBonn #StachnissLab #robotics #PhenoRob #talk
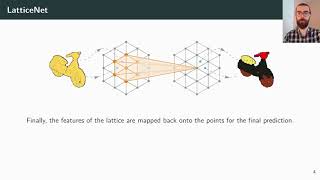
RSS 2020′: LatticeNet: Fast Point Cloud Segmentation Using Permutohedral Lattices
LatticeNet: Fast Point Cloud Segmentation Using Permutohedral Lattices by Radu Alexandru Rosu, Peer Schütt, Jan Quenzel, Sven Behnke Deep convolutional neural networks (CNNs) have shown outstanding performance in the task of semantically segmenting images. However, applying the same methods on 3D data still poses challenges due to the heavy memory requirements and the lack of structured data. Here, we propose LatticeNet, a novel approach for 3D semantic segmentation, which takes as input raw point clouds. A PointNet describes the local geometry which we embed into a sparse permutohedral lattice. The lattice allows for fast convolutions while keeping a low memory footprint. Further, we introduce DeformSlice, a novel learned data-dependent interpolation for projecting lattice features back onto the point cloud. We present results of 3D segmentation on various datasets where our method achieves state-of-the-art performance.
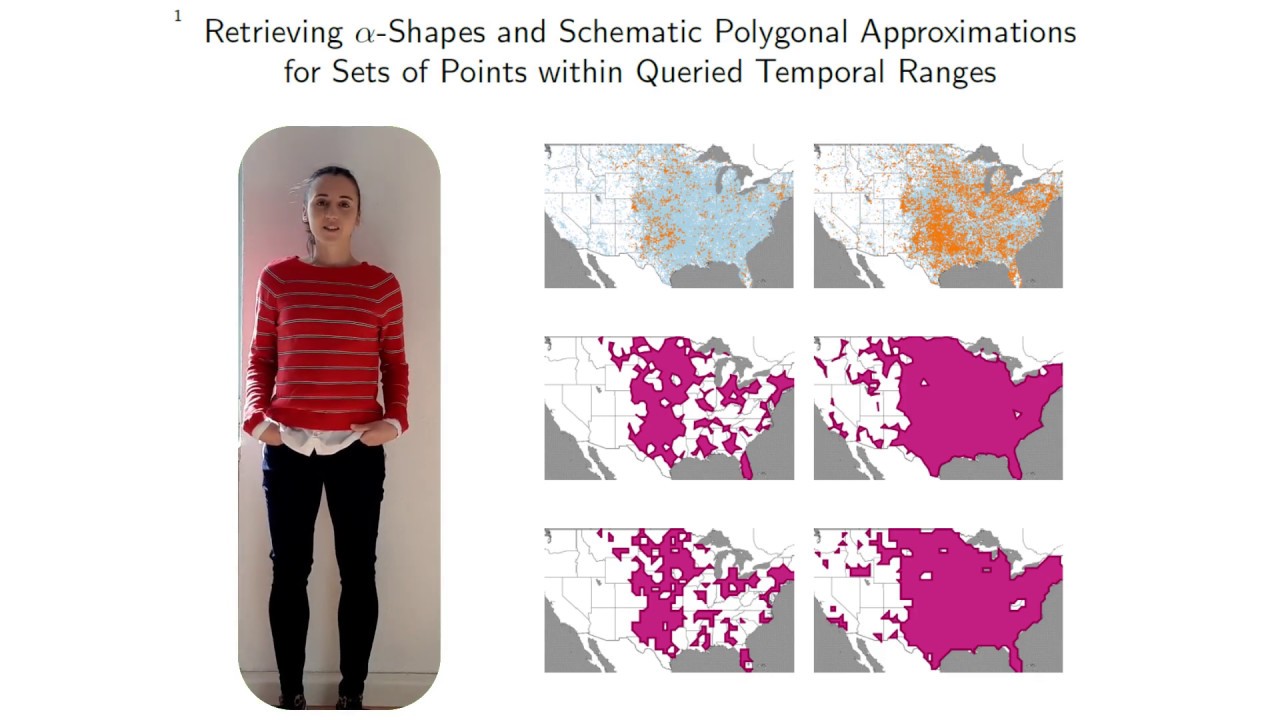
SIGSPATIAL’2019: Retrieving alpha-Shapes and Schematic Polygonal Approximations for Sets of Points..
Retrieving alpha-Shapes and Schematic Polygonal Approximations for Sets of Points within Queried Temporal Ranges by A. Bonerath, B. Niedermann und J.-H. Haunert In Proceedings of the 27th ACM SIGSPATIAL International Conference on Advances in Geographic Information Systems, 2019
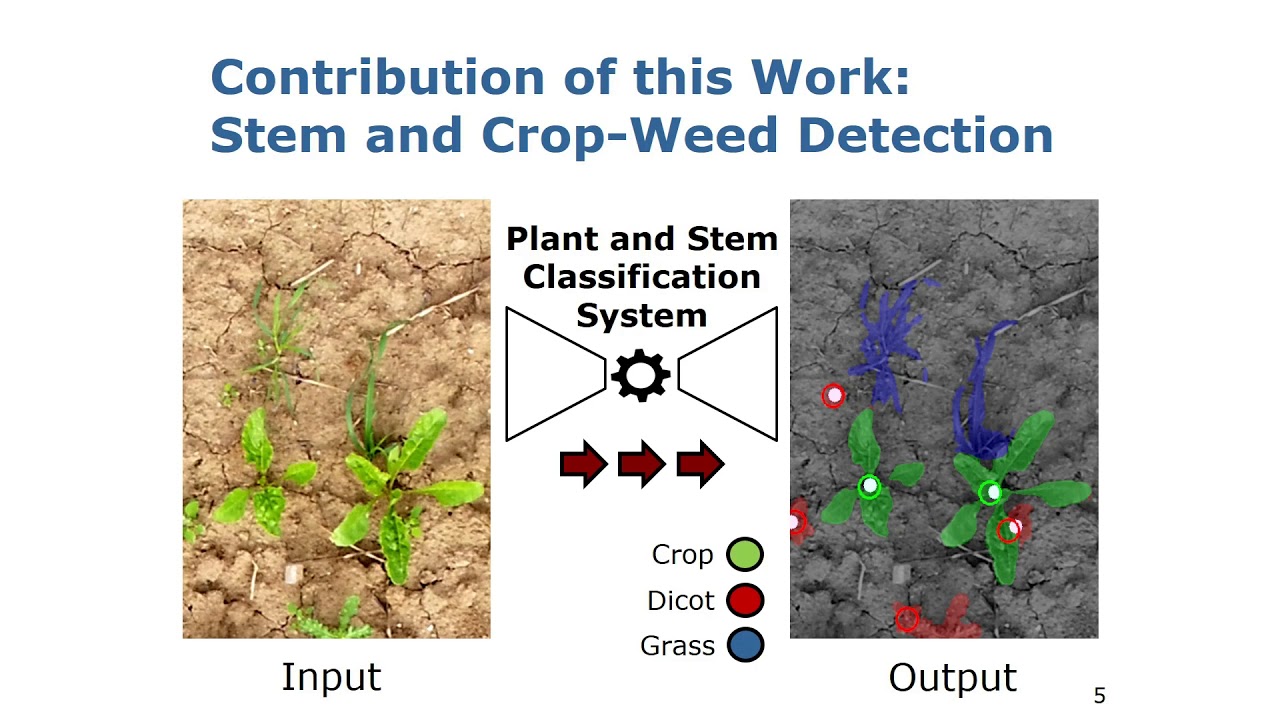
IROS’18: Joint Stem Detection and Crop-Weed Classification for Plant-specific Treatment
Trailer for the paper: Joint Stem Detection and Crop-Weed Classification for Plant-specific Treatment in Precision Farming by P. Lottes, J. Behley, N. Chebrolu, A. Milioto, and C. Stachniss, IROS 2018.
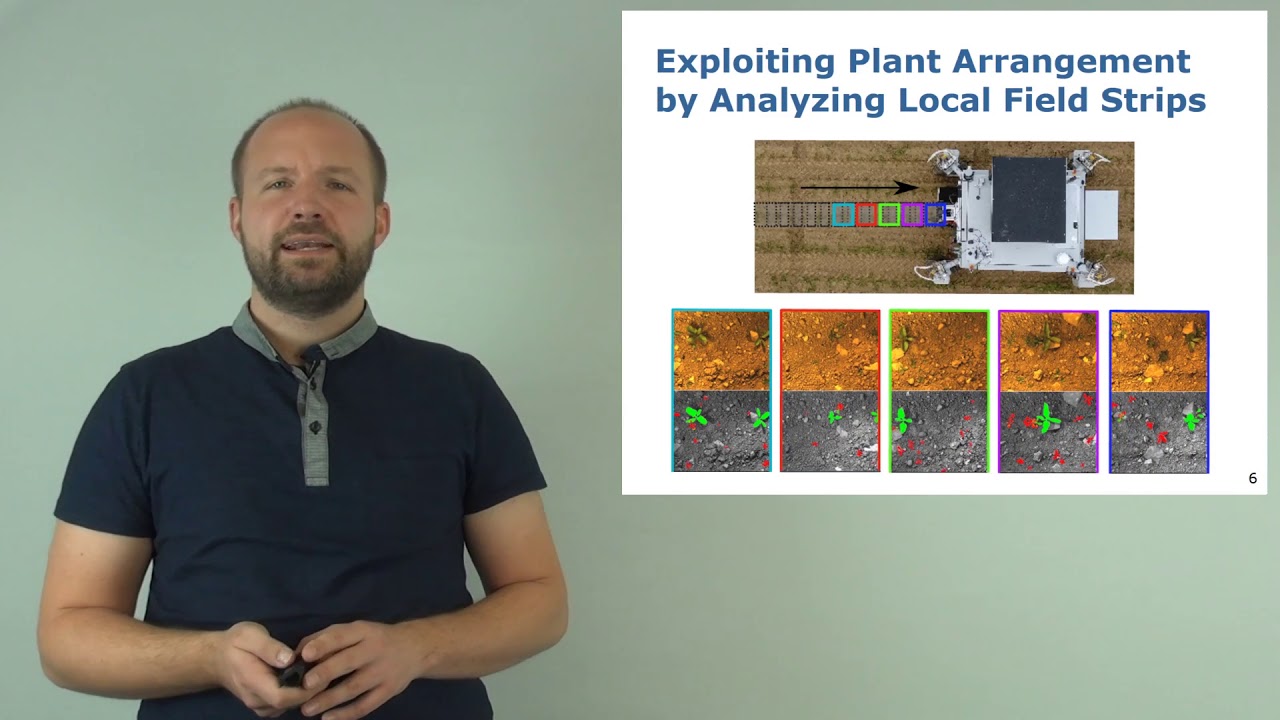
RAL’18: FCNs with Sequential Information for Robust Crop and Weed Detection by Lottes et al.
Trailer for the paper: Fully Convolutional Networks with Sequential Information for Robust Crop and Weed Detection in Precision Farming by P. Lottes, J. Behley, A. Milioto, and C. Stachniss, RAL 2018
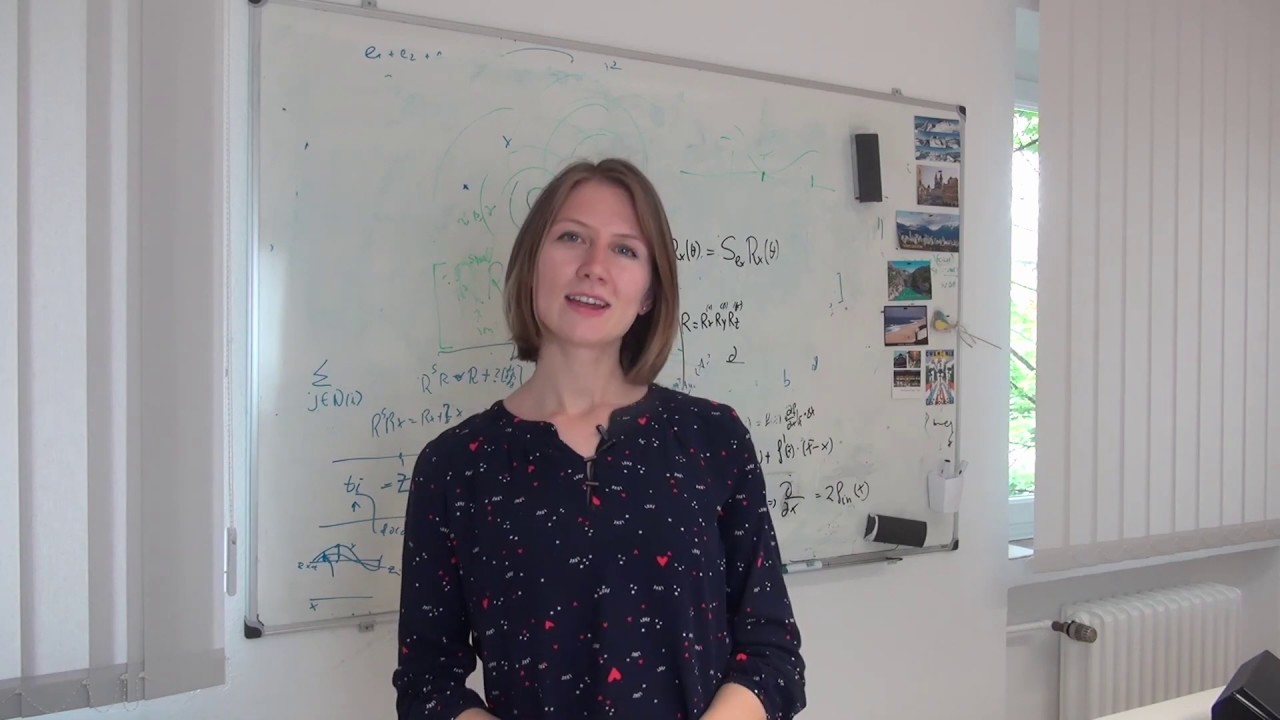
RAL-ICRA’19: Effective Visual Place Recognition Using Multi-Sequence Maps by Vysotska & Stachniss
O. Vysotska and C. Stachniss, “Effective Visual Place Recognition Using Multi-Sequence Maps,” IEEE Robotics and Automation Letters (RA-L) and presentation at ICRA, 2019. PDF: http://www.ipb.uni-bonn.de/wp-content/papercite-data/pdf/vysotska2019ral.pdf

ICRA’19: Actively Improving Robot Navigation On Different Terrains Using GPMMs by Nardi et al.
L. Nardi and C. Stachniss, “Actively Improving Robot Navigation On Different Terrains Using Gaussian Process Mixture Models,” in Proc. of the IEEE Intl. Conf. on Robotics & Automation (ICRA) , 2019. http://www.ipb.uni-bonn.de/wp-content/papercite-data/pdf/nardi2019icra-airn.pdf
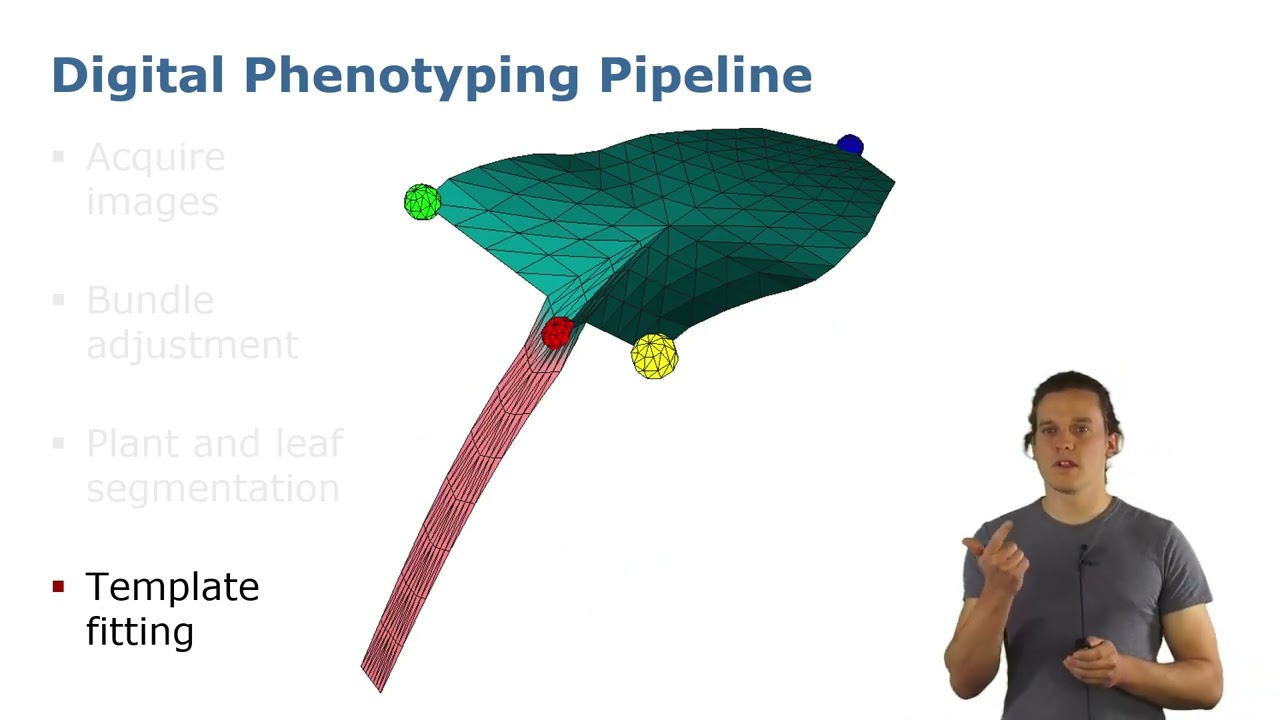
ICRA’22: Precise 3D Reconstruction of Plants from UAV Imagery … by Marks et al.
E. Marks, F. Magistri, and C. Stachniss, “Precise 3D Reconstruction of Plants from UAV Imagery Combining Bundle Adjustment and Template Matching,” in Proc.~of the IEEE Intl.~Conf.~on Robotics & Automation (ICRA), 2022. PDF: https://www.ipb.uni-bonn.de/wp-content/papercite-data/pdf/marks2022icra.pdf #UniBonn #StachnissLab #robotics
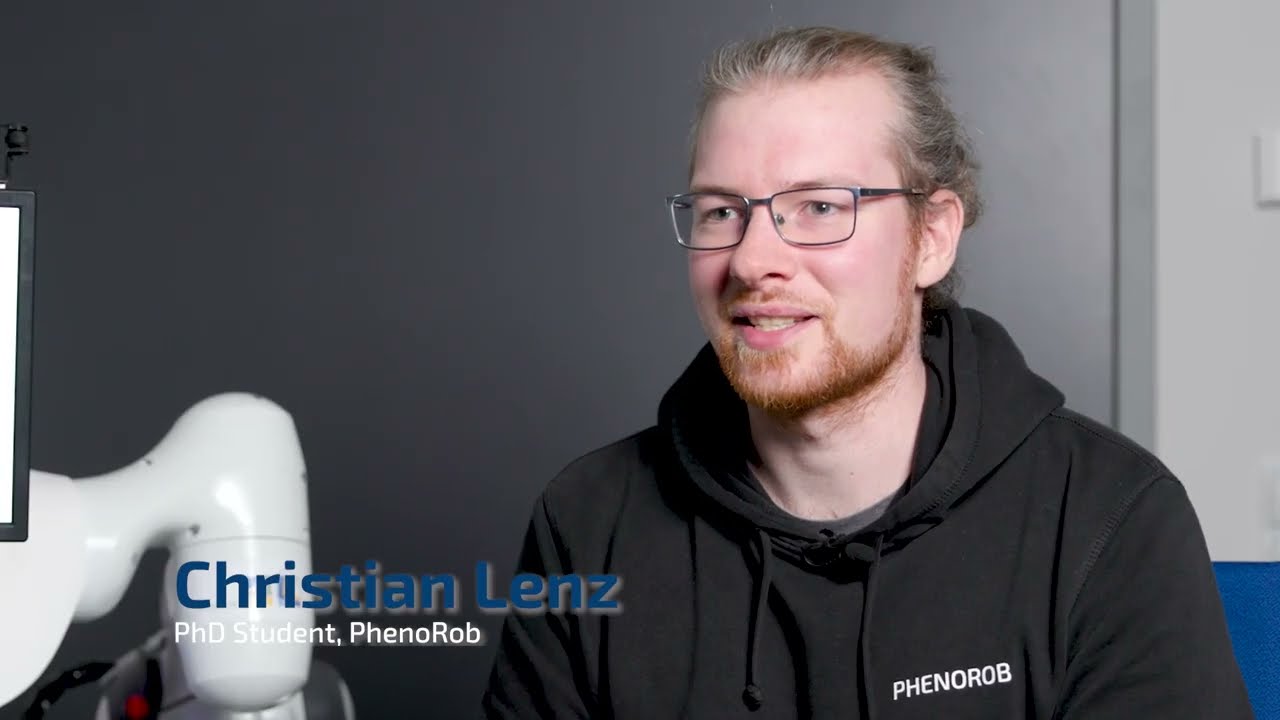
Faces of PhenoRob: Christian Lenz
In Faces of PhenoRob, we introduce you to some of PhenoRob’s many members: from senior faculty to PhDs, this is your chance to meet them all and learn more about the work they do! In this video, you’ll meet Christian Lenz, PhenoRob PhD Student.
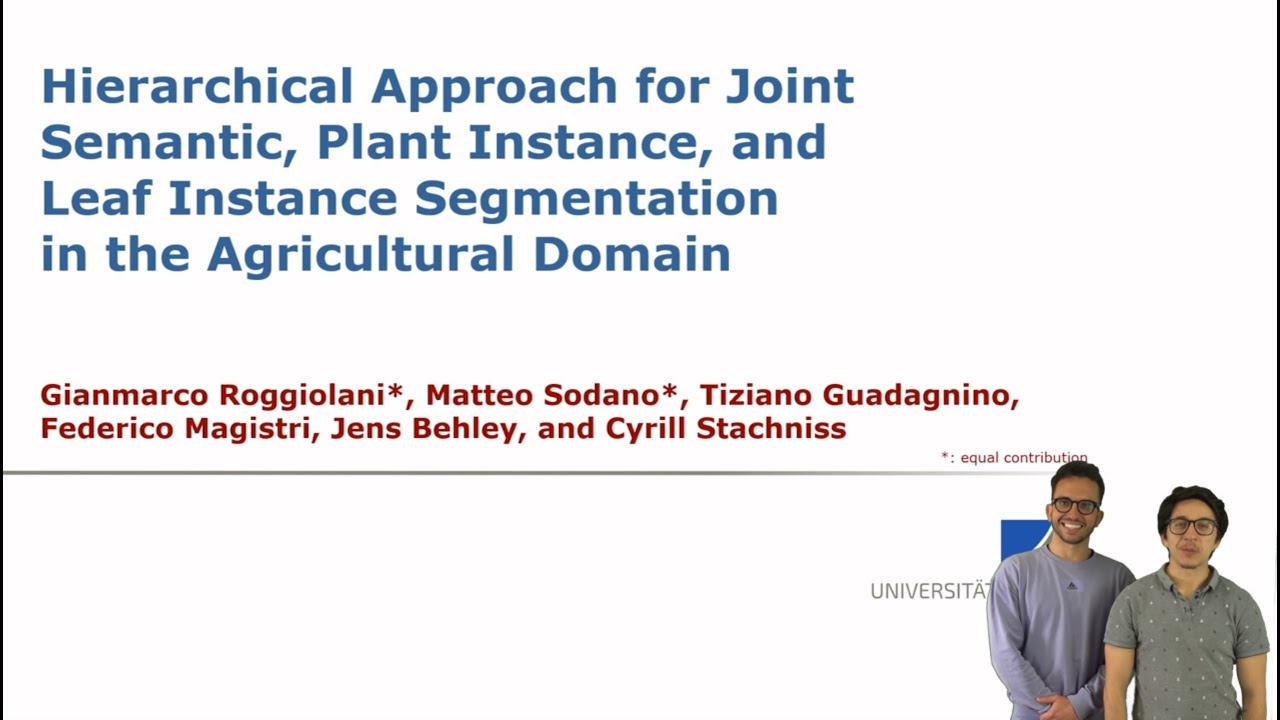
Hierarchical Approach for Joint Semantic, Plant & Leaf Instance Segmentation in the Agricult. Domain
This short trailer is based on the following publication: G. Roggiolani, M. Sodano, F. Magistri, T. Guadagnino, J. Behley, and C. Stachniss, “Hierarchical Approach for Joint Semantic, Plant Instance, and Leaf Instance Segmentation in the Agricultural Domain,” in Proceedings of the IEEE International Conference on Robotics & Automation (ICRA), 2023.
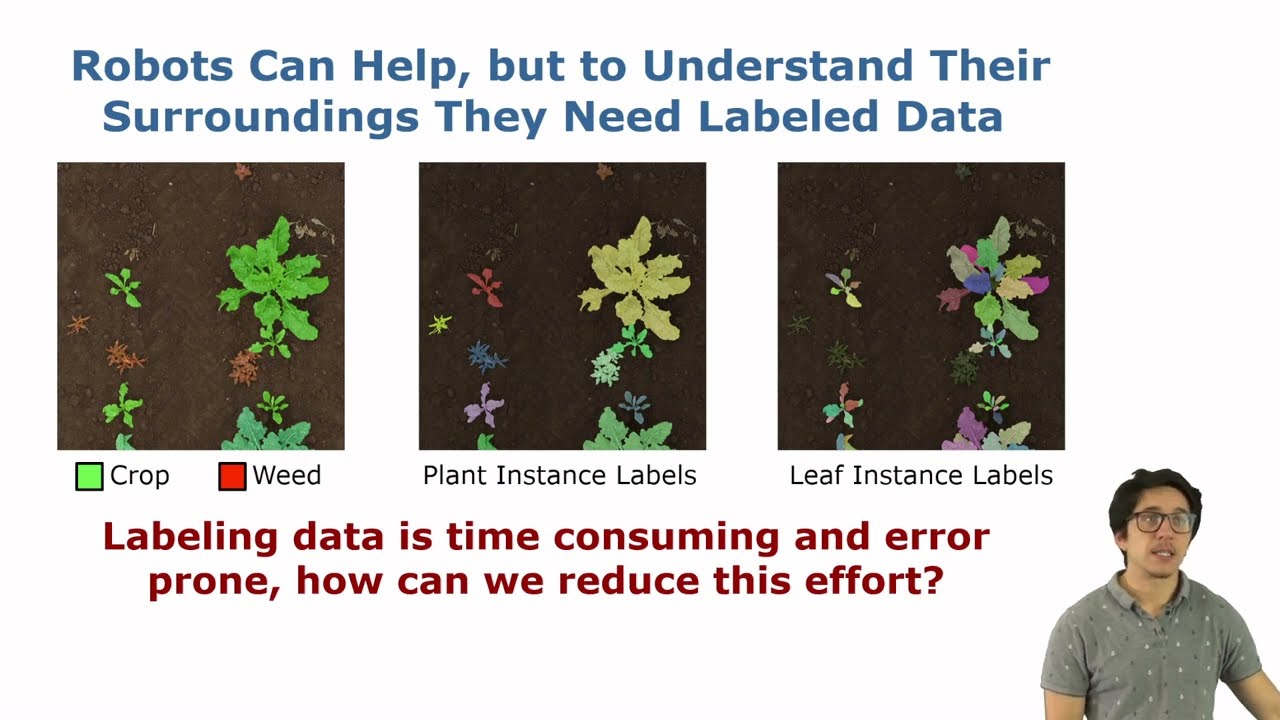
On Domain-Specific Pre-Training for Effective Semantic Perception in Agricult. Robotics (Roggiolani)
This short trailer is based on the following publication: G. Roggiolani, F. Magistri, T. Guadagnino, G. Grisetti, C. Stachniss, and J. Behley, “On Domain-Specific Pre-Training for Effective Semantic Perception in Agricultural Robotics,” Proceedings of the IEEE International Conference on Robotics & Automation (ICRA), 2023.
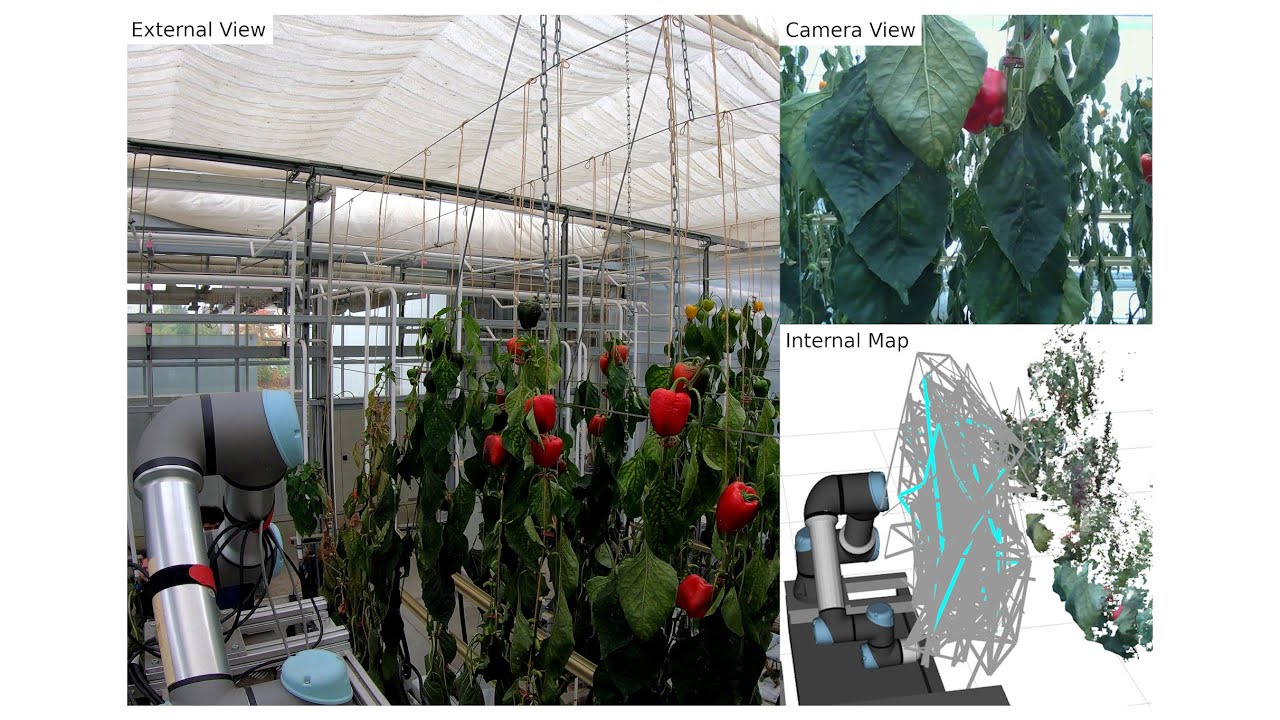
Graph-based View Motion Planning for Fruit Detection
This video demonstrates the work presented in our paper “Graph-based View Motion Planning for Fruit Detection” by T. Zaenker, J. Rückin, R. Menon, M. Popović, and M. Bennewitz, submitted to the International Conference on Intelligent Robots and Systems (IROS), 2023. Paper link: https://arxiv.org/abs/2303.03048 The view motion planner generates view pose candidates from targets to find new and cover partially detected fruits and connects them to create a graph of efficiently reachable and information-rich poses. That graph is searched to obtain the path with the highest estimated information gain and updated with the collected observations to adaptively target new fruit clusters. Therefore, it can explore segments in a structured way to optimize fruit coverage with a limited time budget. The video shows the planner applied in a commercial glasshouse environment and in a simulation designed to mimic our real-world setup, which we used to evaluate the performance. Code: https://github.com/Eruvae/view_motion_planner

NBV-SC: Next Best View Planning based on Shape Completion for Fruit Mapping and Reconstruction
This video demonstrates the work presented in our paper “NBV-SC: Next Best View Planning based on Shape Completion for Fruit Mapping and Reconstruction” by R. Menon, T. Zaenker, N. Dengler and M. Bennewitz, submitted to the International Conference on Intelligent Robots and Systems (IROS), 2023. State-of-the-art viewpoint planning approaches utilize computationally expensive ray casting operations to find the next best viewpoint. In our paper, we present a novel viewpoint planning approach that explicitly uses information about the predicted fruit shapes to compute targeted viewpoints that observe as yet unobserved parts of the fruits. Furthermore, we formulate the concept of viewpoint dissimilarity to reduce the sampling space for more efficient selection of useful, dissimilar viewpoints. In comparative experiments with a state-of-the-art viewpoint planner, we demonstrate improvement not only in the estimation of the fruit sizes, but also in their reconstruction, while significantly reducing the planning time. Finally, we show the viability of our approach for mapping sweet peppers plants with a real robotic system in a commercial glasshouse. Paper link https://arxiv.org/abs/2209.15376
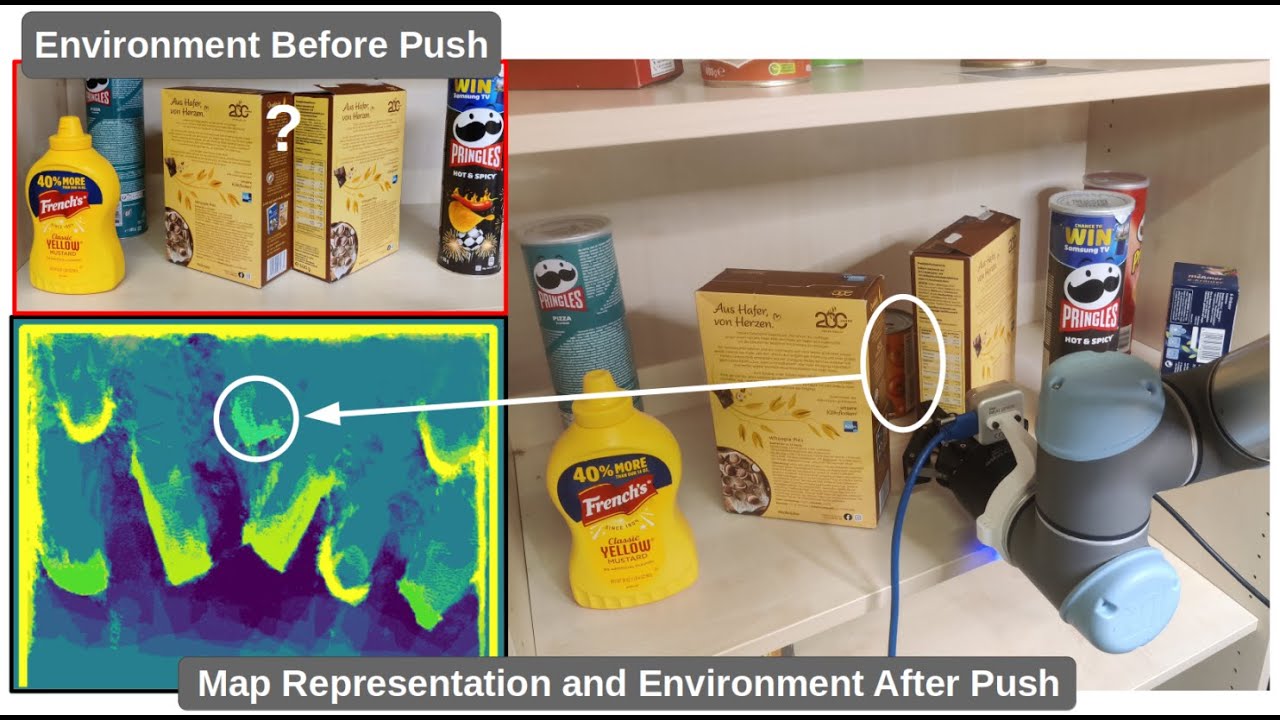
Viewpoint Push Planning for Mapping of Unknown Confined Spaces
This video demonstrates the work presented in our paper “Viewpoint Push Planning for Mapping of Unknown Confined Spaces” by N. Dengler, S. Pan, V. Kalagaturu, R. Menon, M. Dawood and Maren Bennewitz, submitted to the International Conference on Intelligent Robots and Systems (IROS), 2023. Paper link: https://arxiv.org/pdf/2303.03126.pdf The mapping of confined spaces such as shelves is an especially challenging task in the domain of viewpoint planning, since objects occlude each other and the scene can only be observed from the front, thus with limited possible viewpoints. In this video, we show our deep reinforcement learning framework that generates promising views aiming at reducing the map entropy. Additionally, the pipeline extends standard viewpoint planning by predicting adequate minimally invasive push actions to uncover occluded objects and increase the visible space. Using a 2.5D occupancy height map as state representation that can be efficiently updated, our system decides whether to plan a new viewpoint or perform a push. As the real-world experimental results with a robotic arm show, our system is able to significantly increase the mapped space compared to different baselines, while the executed push actions highly benefit the viewpoint planner with only minor changes to the object configuration. Code: https://github.com/NilsDengler/view-point-pushing
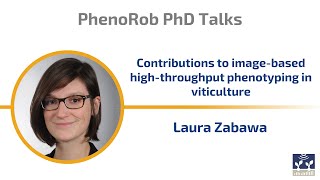
PhenoRob PhD Graduate Talks: Laura Zabawa
Laura Zabawa gives a talk on “Contributions to image-based high-throughput phenotyping in viticulture” after succesfully completing her PhD as part of a PhenoRob partner project. In the PhenoRob PhD Graduate Talks, recent PhenoRob graduates share about their research within the Cluster of Excellence.

PermutoSDF: Fast Multi-View Reconstruction with Implicit Surfaces using Permutohedral Lattices
Neural radiance-density field methods have become increasingly popular for the task of novel-view rendering. Their recent extension to hash-based positional encoding ensures fast training and inference with visually pleasing results. However, density-based methods struggle with recovering accurate surface geometry. Hybrid methods alleviate this issue by optimizing the density based on an underlying SDF. However, current SDF methods are overly smooth and miss fine geometric details. In this work, we combine the strengths of these two lines of work in a novel hash-based implicit surface representation. We propose improvements to the two areas by replacing the voxel hash encoding with a permutohedral lattice which optimizes faster, especially for higher dimensions. We additionally propose a regularization scheme which is crucial for recovering high-frequency geometric detail. We evaluate our method on multiple datasets and show that we can recover geometric detail at the level of pores and wrinkles while using only RGB images for supervision. Furthermore, using sphere tracing we can render novel views at 30 fps on an RTX 3090. The paper, animations, and code is available at https://radualexandru.github.io/permuto_sdf

DawnIK: Decentralized Collision-Aware Inverse Kinematics Solver for Heterogeneous Multi-Arm Systems
This video demonstrates the work presented in our paper “DawnIK: Decentralized Collision-Aware Inverse Kinematics Solver for Heterogeneous Multi-Arm Systems” by S. Marangoz, R. Menon, N. Dengler, and M. Bennewitz, submitted to IEEE-RAS International Conference on Humanoid Robots (Humanoids), 2023. With collaborative service robots gaining traction, different robotic systems have to work in close proximity. This means that the current inverse kinematics approaches do not have only to avoid collisions with themselves but also collisions with other robot arms. Therefore, we present a novel approach to compute inverse kinematics for serial manipulators that take into account different constraints while trying to reach a desired end-effector pose that avoids collisions with themselves and other arms. We formulate different constraints as weighted cost functions to be optimized by a non-linear optimization solver. Our approach is superior to a state-of-the-art inverse kinematics solver in terms of collision avoidance in the presence of multiple arms in confined spaces with no collisions occurring in all the experimental scenarios. When the probability of collision is low, our approach shows better performance at trajectory tracking as well. Additionally, our approach is capable of simultaneous yet decentralized control of multiple arms for trajectory tracking in intersecting workspace without any collisions. Paper link https://arxiv.org/abs/2307.12750
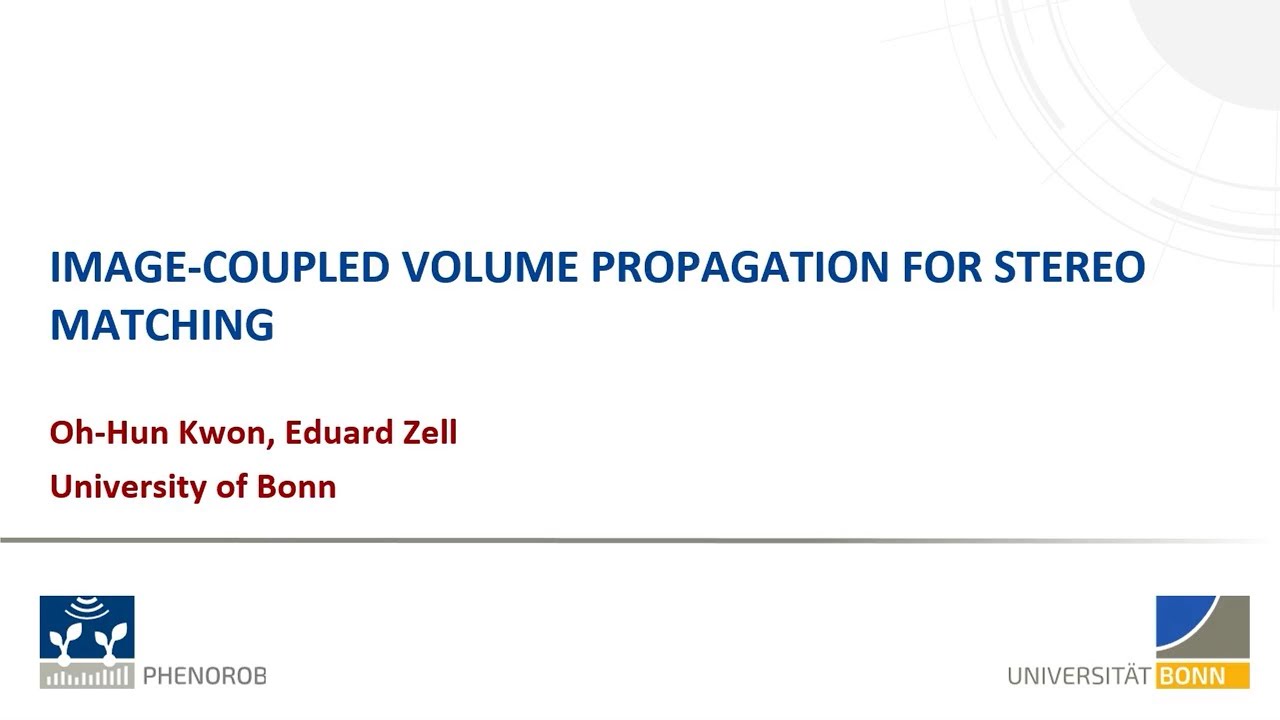
Image-Coupled Volume Propagation for Stereo Matching by Oh-Hun Kwon and Eduard Zell
This trailer video is based on following publication: O. Kwon and E. Zell, “Image-Coupled Volume Propagation for Stereo Matching,” in 2023 IEEE International Conference on Image Processing (ICIP), 2023, pp. 2510-2514. doi:10.1109/ICIP49359.2023.10222247